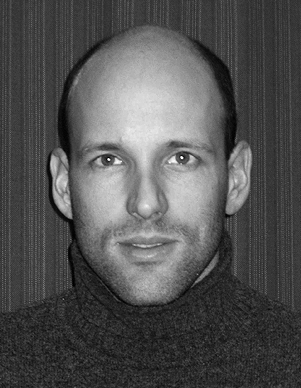
CMR Scientist
Image processing, numerical modelling, machine learning
Publications
2022
![[DOI]](http://vis.test.uib.no/wp-content/plugins/papercite/img/external.png)
@article{Hodneland2022Fully,
title = {Fully Automatic Whole-Volume Tumor Segmentation in Cervical Cancer},
author = {Hodneland, Erlend and Kaliyugarasan, Satheshkumar and Wagner-Larsen, Kari Str{\o}no and Lura, Nj{\aa}l and Andersen, Erling and Bartsch, Hauke and Smit, Noeska and Halle, Mari Kylles{\o} and Krakstad, Camilla and Lundervold, Alexander Selvikv{\aa}g and Haldorsen, Ingfrid S},
year = 2022,
journal = {Cancers},
publisher = {MDPI},
volume = 14,
number = 10,
pages = 2372,
doi = {10.3390/cancers14102372},
url = {https://pubmed.ncbi.nlm.nih.gov/35625977/},
images = "images/Hodneland-2022-Fully.PNG",
thumbnails = "images/Hodneland-2022-Fully.PNG",
project = {ttmedvis}
}
2020
![[PDF]](http://vis.test.uib.no/wp-content/plugins/papercite/img/pdf.png)
![[DOI]](http://vis.test.uib.no/wp-content/plugins/papercite/img/external.png)
![[YT]](http://vis.test.uib.no/wp-content/papercite-data/images/youtube.png)
@article{RadEx,
author = {M\"{o}rth, E. and Wagner-Larsen, K. and Hodneland, E. and Krakstad, C. and Haldorsen, I. S. and Bruckner, S. and Smit, N. N.},
title = {RadEx: Integrated Visual Exploration of Multiparametric Studies for Radiomic Tumor Profiling},
journal = {Computer Graphics Forum},
volume = {39},
number = {7},
year = {2020},
pages = {611--622},
abstract = {Better understanding of the complex processes driving tumor growth and metastases is critical for developing targeted treatment strategies in cancer. Radiomics extracts large amounts of features from medical images which enables radiomic tumor profiling in combination with clinical markers. However, analyzing complex imaging data in combination with clinical data is not trivial and supporting tools aiding in these exploratory analyses are presently missing. In this paper, we present an approach that aims to enable the analysis of multiparametric medical imaging data in combination with numerical, ordinal, and categorical clinical parameters to validate established and unravel novel biomarkers. We propose a hybrid approach where dimensionality reduction to a single axis is combined with multiple linked views allowing clinical experts to formulate hypotheses based on all available imaging data and clinical parameters. This may help to reveal novel tumor characteristics in relation to molecular targets for treatment, thus providing better tools for enabling more personalized targeted treatment strategies. To confirm the utility of our approach, we closely collaborate with experts from the field of gynecological cancer imaging and conducted an evaluation with six experts in this field.},
pdf = "pdfs/Moerth-2020-RadEx.pdf",
images = "images/Moerth-2020-RadEx.jpg",
youtube = "https://youtu.be/zwtDzwwX790",
thumbnails = "images/Moerth-2020-RadEx-thumb.jpg",
project = "ttmedvis",
doi = {10.1111/cgf.14172}
}
2018
![[PDF]](http://vis.test.uib.no/wp-content/plugins/papercite/img/pdf.png)
@MISC {Smit18MMIV,
author = "N. N. Smit and S. Bruckner and H. Hauser and I. Haldorsen and A. Lundervold and A. S. Lundervold and E. Hodneland and L. Oltedal and K. Specht and E. R. Gruner",
title = "Research Agenda of the Mohn Medical Imaging and Visualization Centre in Bergen, Norway",
howpublished = "Poster presented at the EG VCBM workshop 2018",
month = "September",
year = "2018",
abstract = "The Mohn Medical Imaging and Visualization Centre (MMIV) was recently established in collaboration between the University of Bergen, Norway, and the Haukeland University Hospital in Bergen with generous financial support from the Bergen Research Foundation (BFS) to conduct cross-disciplinary research related to state-of-the-art medical imaging, including preclinical and clinical high-field MRI, CT and hybrid PET/CT/MR.The overall goal of the Centre is to research new methods in quantitative imaging and interactive visualization to predict changes in health and disease across spatial and temporal scales. This encompasses research in feature detection, feature extraction, and feature prediction, as well as on methods and techniques for the interactive visualization of spatial and abstract data related to and derived from these features.With special emphasis on the natural and medical sciences, the long-term goal of the Centre is to consolidate excellence in the interplay between medical imaging (physics, chemistry, radiography, radiology), and visualization (computer science and mathematics) and develop novel and refined imaging methods that may ultimately improve patient care. In this poster, we describe the overall research agenda of MMIV and describe the four core projects in the centre.",
pdf = "pdfs/smit2018posterabstract.pdf",
images = "images/MMIVPoster.png",
thumbnails = "images/MMIVPoster.png",
location = "Granada, Spain",
project = "VIDI"
}
2016
![[PDF]](http://vis.test.uib.no/wp-content/plugins/papercite/img/pdf.png)
![[DOI]](http://vis.test.uib.no/wp-content/plugins/papercite/img/external.png)
![[YT]](http://vis.test.uib.no/wp-content/papercite-data/images/youtube.png)
@INPROCEEDINGS {Stoppel-2016-GIR,
author = "Sergej Stoppel and Erlend Hodneland and Helwig Hauser and Stefan Bruckner",
title = "Graxels: Information Rich Primitives for the Visualization of Time-Dependent Spatial Data",
booktitle = "Proceedings of VCBM 2016",
year = "2016",
pages = "183--192",
month = "sep",
abstract = "Time-dependent volumetric data has important applications in areas as diverse as medicine, climatology, and engineering. However, the simultaneous quantitative assessment of spatial and temporal features is very challenging. Common visualization techniques show either the whole volume in one time step (for example using direct volume rendering) or let the user select a region of interest (ROI) for which a collection of time-intensity curves is shown. In this paper, we propose a novel approach that dynamically embeds quantitative detail views in a spatial layout. Inspired by the concept of small multiples, we introduce a new primitive graxel (graph pixel). Graxels are view dependent primitives of time-intensity graphs, generated on-the-fly by aggregating per-ray information over time and image regions. Our method enables the detailed feature-aligned visual analysis of time-dependent volume data and allows interactive refinement and filtering. Temporal behaviors like frequency relations, aperiodic or periodic oscillations and their spatial context are easily perceived with our method. We demonstrate the power of our approach using examples from medicine and the natural sciences.",
pdf = "pdfs/Stoppel-2016-GIR.pdf",
images = "images/Stoppel-2016-GIR.jpg",
thumbnails = "images/Stoppel-2016-GIR.png",
youtube = "https://www.youtube.com/watch?v=UsClj3ytd0Y",
doi = "10.2312/vcbm.20161286",
event = "VCBM 2016",
keywords = "time-dependent data, volume data, small multiples",
location = "Bergen, Norway"
}
2014
![[DOI]](http://vis.test.uib.no/wp-content/plugins/papercite/img/external.png)
![[VID]](http://vis.test.uib.no/wp-content/papercite-data/images/video.png)
@ARTICLE {Angelelli14Interactive,
author = "Paolo Angelelli and Steffen Oeltze and Cagatay Turkay and Judit Haasz and Erlend Hodneland and Arvid Lundervold and Astri Johansen Lundervold and Bernhard Preim and Helwig Hauser",
title = "Interactive Visual Analysis of Heterogeneous Cohort Study Data",
journal = "Computer Graphics and Applications, IEEE",
year = "2014",
volume = "PP",
number = "99",
pages = "1-1",
abstract = "Cohort studies are used in medicine to enable the study of medical hypotheses in large samples. Often, a large amount of heterogeneous data is acquired from many subjects. The analysis is usually hypothesis-driven, i.e., a specific subset of such data is studied to confirm or reject specific hypotheses. In this paper, we demonstrate how we enable the interactive visual exploration and analysis of such data, helping with the generation of new hypotheses and contributing to the process of validating them. We propose a data-cube based model which allows to handle partially overlapping data subsets during the interactive visualization. This model enables the seamless integration of the heterogeneous data, as well as the linking of spatial and non-spatial views on these data. We implemented this model in an application prototype, and used it to analyze data acquired in the context of a cohort study on cognitive aging. In this paper we present a case-study analysis of selected aspects of brain connectivity by using a prototype implementation of the presented model, to demonstrate its potential and flexibility.",
vid = "vids/angelelli14CohortExplorer.wmv",
images = "images/angelelli14Cohort.png",
thumbnails = "images/angelelli14Cohort.png",
doi = "10.1109/MCG.2014.40",
url = "http://dx.doi.org/10.1109/MCG.2014.40"
}